MPI Central
***Disclaimer***
The MPI Assist Tool should only be used after a conceptual framework has been built with solid justification or during national consultations.
Before exploring the MPI Central tab, please refer to training modules available on the multi dimensional poverty portal. These modules will provide you with more extensive information on the rationale of the MPI as well as a detailed technical guide on calculating the MPI. Member states may consider the following participatory approach.

Building a National MPI: The 8 Steps of the MPI Central Tab
Find the MPI Central tab to begin:

Step 1 Select survey
Choose country and select the year
You can select from the predefined frameworks on the right hand side, or continue with a new one by clicking Build new framework.
Only years with available surveys for the selected country will appear.
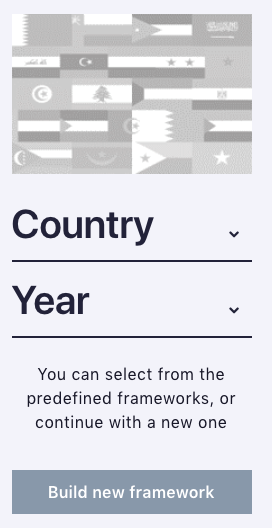
If you make any changes in the Indicators tab, make sure you click fetch next to the indicator to reduce the overall computational time in the MPI Central tab.
Step 2 Define the dimensions and group indicators
The screen will have boxes for the dimensions to be defined and a list of predefined indicators to select from.

Click on the blue box to name your first dimension.
We will start with the Health dimension under the 2020 Revised Arab MPI framework.

You can rename your dimension at any time on this screen.

All available indicators are shown in the box on the right.
If you hover over an indicator you can access the default definition of that indicator.

Drag and drop indicators to group them under the relevant dimension.
Clickat the top of the dimensions
box to add a new dimension.
We will add the dimensions under the 2020 Revised Arab MPI.
For the Health dimension, simply drag and drop the relevant indicators: child mortality, child nutrition and early pregnancy.

To deselect an indicator, simply drag it out of the dimension box/group, back into the indicator box.
You can click the delete button to erase all indicators in that dimension.
For the second dimension, add a new dimension and call it Education. Again, drag and drop the relevant indicators: school attendance, age schooling gap and educational attainment.

For the Housing dimension, select and group several relevant indicators including overcrowding and type of dwelling (roof and floor).

For the Services dimension, select and group several relevant indicators including improved drinking water, improved sanitation and electricity.

For the Assets dimension, select and group several relevant indicators including communications assets, mobility assets and livelihood assets.

After grouping indicators by dimension, you will have the option to set the deprivation cut-off points for each indicator.
When you are ready to set the cut offs Click
Step 3: Deprivation cut-offs
A grey box in the indicator definition allows you to change the cut-offs for each indicator.
For example, for the education dimension, the cut-off point for the educational attainment indicator is changeable.

Next to the indicator name you will find the results for each indicator: the red shows total deprived and the green shows total non-deprived.

If you change the cut-off point, click the refresh button to refresh the results for total deprived and total non-deprived.

When satisfied with the deprivation cut-off points, click to set the weights for the indicators and dimensions
Step 4: Set the weights for the indicators within each dimension
In this step, you will be prompted to specify the weights by indicator and by dimension.
→The total percentage of all dimensions must equal 100.
→The overall percentage of indicators within each dimension must equal 100.
By default, the various dimensions and the various indicators within each dimension are given equal weights.

- For the dimensions, weights can be changed by sliding the red bar or by inputting the fraction below in the blue box on the left.
-
For the indicators, weights can be changed by sliding the red bar or by inputting the fraction
below on the right.
The slider accepts weights with two decimal point. For more accurate positioning, you can click on the slider and use the right and left arrows of the keyboard to move it up or down. You may also insert fractions or exact numbers in a box next to the slider.
- The validation of the overall weights on dimensions will show on the bottom left.
-
The weights for indicators will show on the right side near each grouping of indicators.
To help you make sure the weights add up to 100, the total weights within each dimension is displayed at the top of the blue dimension box. When the weights total 100 per cent, the number '100%' is shown in green and you are ready to move on to the next dimension. If the weights do not total 100 per cent, the figure will be shown in red and a change will need to be made to bring them in line.
-
Weights can be reset at any time. To do so, click
Click to proceed to step 5!
Step 5: Framework Summary
A table is shown providing a comprehensive summary of the chosen framework including the dimensions, indicators, weights, cut-offs, headcount ratio and definitions for each indicator.
The adjusted cut-offs are reflected in the indicator definitions.

Clickto submit the MPI for live calculation.
Please give this step some time. At this stage MAT is accessing the raw household survey data and applying all the cut-off rules to calculate a binary record of deprivation and non-deprivation for 60,000-150,000 individual records depending on the country. MAT then generates the overall deprivation scores using the weights specified per indicator and per dimension. Once the calculation is complete, MAT will provide the distribution of deprivations.
Step 6: Set the poverty cut-off
Upon submitting, a similar graph will appear showing the deprivation distribution in the country you selected.

The first box on the left hand-side shows the total number of individuals in the survey excluding those with missing values.
There are three lines presented on this graph:
- Vulnerability Cut-off (Green line)
- Poverty Cut-off (Yellow line)
- Extreme poverty Cut-off (Red line)
The yellow line determines the output for the results page, the red and green lines provide additional information on destitution and extreme poverty. (Users will be able to access the additional two result pages for destitution and extreme poverty in Version 2 (V2) of MAT).
By sliding the yellow line, you will see the headcount change in the yellow middle box. This reflects the number of people with a deprivation score greater than the cut-off specified. The farther right the yellow line (closer to the red line), the higher the deprivation score but the lower the poverty headcount. Similarly, the farther left the yellow line (closer to the green line), the lower the deprivation score but the higher the poverty headcount.
Similarly, the red line reflects the extreme poverty cut-off and headcount (in the red box). As for the vulnerability headcount, based on the green line, it reflects the number of persons below the green line and above the yellow poverty line (in the green box). These individuals are vulnerable to falling below the poverty line if they become deprived in at least one additional indicator.
The order of the 3 lines has an analytical significance, MAT will prompt an error if the three lines are not in the correct order.
Finally, the real distribution of deprivations can be revealed by switching-on the 'show histogram' on the top right corner.
Hovering over any point in the graph will give you the number of individuals with that deprivation score (not a cumulative headcount).

According to your selected cut-offs, the rest of the results can be calculated.
Click Computing the results will take around one
minute.
Step 7: Specify the outputs
General output
The results page will present tabs pertaining all results
The results screen shows a summary of the MPI, the headcount ratio and the average intensity of deprivation. You will find several collapsable sections of available outputs (tables, graphs, etc.)
Click on the icon on the top right corner of each output to save as an image or download the data view as an excel.
Find and click on the three dots on the top right corner of the result page to either expand or collapse all results tabs.

The results page also shows the relative contribution of the different dimensions to the MPI

Output by socio-demographic variables
The MPI framework can be broken down by socio-demographic properties. For example, you may break down the MPI by rural/urban.

In this case, the disaggregated evidence can be analysed to show the contribution of various indicators and dimensions to poverty in rural and urban areas, separately. This provides important information for policymakers and policy analysis.

Cramer’s V and Redundancy Measure R0
Click on read more to better understand the two measures
Use the slider on the side to highlight association between two indicators
You can hover over the boxes to retain their value
Cramer’s V measures the level of association between two categorical values

Redundancy Measure R0 presents the matches between deprivations as a ration of the minimum marginal deprivation rates

Step 8: Save/Print
The results of a framework can be saved or printed.
Click "Save" to keep the entire framework for use in later comparisons. You will be prompted to name your saved framework.
Once saved, you will be redirected to step 1 where you can see the list of saved frameowrks. You can click "Load" to view your framework, or you can click
"Load and Edit" to introduce changes and save it under another name.
Please note, any saved framework will be storing an image of all indicators as defined at the time of saving.
Click "print" to print the entire results page or save it as a PDF.
Indicator Builder
Getting started
Find the Indicators tab to begin:

This tab is a space dedicated for technical persons that are familiar with the data and are ready to build the relevant poverty indicators, in the MAT. The built indicators herein will be accessible to be used in the MPI Central tab.
Select Survey
Select the country and year from the Select Survey drop-down menu:

The screen will be empty if the survey is newly uploaded, or it will show a list of pre-set indicators and their definitions built by other users, validated by ESCWA experts and made public to users. Most pre-set indicators follow either the "Revised Arab MPI Framework" or the "National MPI Framework", when available. The definitions include the rules and variables used to construct the indicator.
Revised Arab MPI Framework
All indicator examples given in this manual are based on the Revised Arab MPI.

Single Rule Indicators
Single Rule indicators are the simplest form of building an indicator where only one single variable is used to define the deprivation versus the non -deprivation
To illustrate this, let us take the Electricity indicator as an example:
Electricity Indicator
To begin, click on
Name your indicator in the Title box and write the definition for your indicator in the Definition box. After naming and defining general information on the indicator, find the Rules tab to continue:

Click on
In this example the variable called electricity can be found in the HH file as such:
Select the source file: HH (Household)

Select the variable: From the drop-down list, select the electricity variable:

Identify the variables of interest: In this example the variable is called "electricity".
- The variable option will have a drop-down list including a search bar. You may search by variable name or any word in its label/code.
- Next to the variable name a percentage appears between brackets: this is the per cent of data points present for each variable.
- At this point MAT will create a deprivation variable for this indicator where all HH who do not have electricity will be deprived with a value of 1, and the others will be non-deprived with a value of zero, unless they have a missing value which will be kept as missing in the created variable.
Select the value: Choose No. This will assume that if the household has electricity then the HH is not deprived.

Click
Click
If you click close without saving, the indicator will not be saved.
Composite Indicators
The next two examples will cover composite indicators in which the user can choose an 'AND' / 'OR' option. An indicator can be based on one or more rules, and each rule can include one or more conditions.

The logic here is to follow the definition and include all elements contained in it. Some definitions include an 'or' option, and some definitions include an 'and' option.

Using the AND operator: HH is considered deprived if HH is deprived in both var(1) and var(2).
Using the OR operator: HH is considered deprived if HH is deprived in either var(1) or var(2).
If you want to add an AND operator to your variable click on the green plus (+) sign to the right of the indicator allows you to add an 'and' operator/condition.
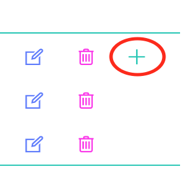
If you want to add an OR operator to your variable click on on the left.
Mobility Assets Indicator (AND operator)
Click on
Name your indicator in the Title box and write the definition for your indicator in the Definition box. After naming and defining general information on the indicator, find the Rules tab to continue:

Let us look at the Mobility Assets indicator and its definition:
The household has no car/truck, motorbike or bicycle.
In order to take into account that the household can have either of the options, we must input all available options from within the definition: car/truck, motorbike and bicycle.
Click on
Select the source: HH (Household)

Select the variable: From the drop-down list, find and select the options from within the definition. To begin select Car/truck:

Select the value: Choose no. This will assume that if the household has a car/truck then the HH is not deprived.

Click
You will notice that this step allows you to add an 'and' operator as part of the definition for the same indicator.
The logic here is to follow the definition and include all elements contained in it. Some definitions include an 'or' option, and some definitions include an 'and' option.
Since the definition states that the HH is deprived if no HH member has a car/truck or motorbike (in other words, no HH member has a car/truck and no HH member has a motorbike), you will need to add the motorbike option from the drop-down list of variables.
Click on the green plus (+) sign to the right of the indicator will allow you to add an 'and' option.
Click
Since the definition states that the HH is not deprived if any HH member has a car/truck, motorcycle/scooter or bicycle , follow the same steps for the additional variables: in this case repeat the steps for motorbike/scooter and bicycle. Select the source, select the variable and select the deprivation value. The final results will show as follow:

Now, you can see that all options are included as stated in the definition.
Once satisfied with your indicator, click
Click
Once you click fetch, the results will show as follow:

Click on the fetch button to see results and reduce computation time

Improved Sanitation Indicator (Categorical/OR Operator)
Click on
Name your indicator in the Title box and write the definition for your indicator in the Definition box. After naming and defining general information on the indicator, find the Rules tab to continue:

Let us look at the Improved Sanitation indicator and its definition
"Deprived if the household does not have access to improved sanitation or it is improved but shared with other households"
Click on
Select the source: HH (Household)

Select the variable: From the drop-down list, select the variable for the Type of toilet:

Since this is qualitative and categorical, skip the operator and user-defined value option.
Select the value: The drop down list will present options related to sanitation that would indicate deprivation of a HH.
Note: You may select multiple options from the drop down list:

Click
Let us look at the definition again:
"Deprived if the household does not have access to improved sanitation OR it is improved but shared with other households"
Click on to produce the OR operator
Select the source: HH

Select the variable:From the drop-down list, select the variable for Shared Toilet

skip the user defined value and operator
Select the value: Yes

Click
Click
The results page should appear as follows:

Quantitative Rules
Some variables that you will use to build indicators are not categorical. Such numeric variables will not present any labels. In such a case, you will need to set a numeric cut-off, as illustrated in the examples below.
Overcrowding Indicator (Quantitative Rule)
Click
Name your indicator in the Title box and write the definition for your indicator in the Definition box. After naming and defining general information on the indicator, find the Rules tab to continue:
Let us look at the overcrowding indicator and its definition:
HH is deprived if the HH has 3 persons or more, aged 10+ years per sleeping room.
Find and click the Rules tab for the indicator.
Click on
Select the source: HH (Household)

Select the variable: From the drop-down list, choose Crowding

Select the operator: Following the definition, we want to set deprivation starting at 3 persons or more. As such, set the operator to match the definition as greater than or equal to.

Select the value: In the value field, type "3", or use the arrows that appear when hovering over the field.

Click on
Click on to save the indicator.
Placeholders
Placeholders allow the user to change the cut-off point of an Indicator in the MPI Central tab.

Placeholders are set within the definition; you can choose what number a user can change in the MPI Central tab. We call this setting a placeholder. You will need to include it in the indicator display box once the rules have been set.
In the previous example you created the Overcrowding Indicator, you will now continue editing that same indicator by adding a user defined value to it.
Overcrowding Indicator, continued (Quantitative Placeholder)
Find your Overcrowding Indicator in the list of indicators and click the edit button

Click the edit button

From the definition, we can understand that a HH that has 3 persons or more aged 10+ years sleeping in the same room is considered deprived.
In this example, the user can change the the number of people in a HH sleeping in the same room to adjust the deprivation cut-off. This will change the deprivation score accordingly. Let's try this out!
Find the Rules tab
Click the edit button
Click/switch on the user defined value. This feature will allow the MPI central to have users change the cut-off of an indicator.

Select the default value: Set the default value at 3 since the definition states 3 persons or more

Select the placeholder: Since this the first and only placeholder you are setting for this indicator then please choose {1}

Placeholder {1} should be added in the indicator display instead of the number '3'. To do so, select the general info tab and scroll down to the Indicator Display. Make sure to include the placeholder in its correct location for it to be reflected in the MPI central tab.
Please differentiate between the definition box and the indicator display box.

Click on
Click
If you click close without saving, no changes will be saved.
Defining Deprivation at the Individual Level (ALL/ANY)
Unlike the HH variables shown in the examples above, individual indicators need additional specification for whether the deprivation rule should apply to ALL HH members or ANY one member of the HH. The ALL/ANY options do not apply in HH variables such as those in the previous examples
In every indicator general info tab you will find: Any one person in the household / All persons in the household

Let us take an example:
Assuming a Household (HH) has 4 children and you are using the School Attendance indicator:
HH is deprived if any of the four children in the household have not attended school.
You can define the indicator in multiple ways. Please choose the appropriate option based on your preffered definition:
→ The HH is deprived if ANY one of the 4 children have not attended school
or
→ The HH is deprived if ALL 4 children have not attended school
The back end of MAT will take your ALL/ANY specification to expand the deprivation status at the HH level. The table below shows the different output scenarios.

Case 1 is a HH unit including three individuals, one that is not deprived (ND), one that is deprived (D) and the other undisclosed. If the definition states that:
HH is deprived if ANY one of the three members is deprived
→ then the HH will be considered deprived since there is one HH member that is deprived
HH is deprived if ALL three members are deprived
→ then the HH is not deprived since there is only one HH member that is deprived and not ALL
Early Pregnancy Indicator (ANY - Placeholder/AND operator)
Click on
Name your indicator in the Title box and write the definition for your indicator in the Definition box. After naming and defining general information on the indicator, find the Rules tab to continue:

Let us look at the Early Pregnancy indicator and its definition:
HH is deprived if any woman aged 15-24 in the household gave birth before the age of 18
From the definition, we can understand that the HH is deprived if ANY woman aged 15-24 gave birth before the age of 18. As such, select the Any option since it matches the definition

Click on
Select the source: WN (Women surveys)

Select the variable: Ever given birth

Skip the operator and user defined value
Select value: yes

Then click
Click the green plus (+) sign to the right of the indicator to add an ' 'AND' option:

The definition states that any woman aged 15-24 who gave birth before the age of 18 is considered deprived.
Pay attention to the data structure you are using. In this specific survey, the women file module interviews only women aged between 15-49. In this case, MAT will treat all other individuals beyond this group (outside the women file) as non-deprived by default. Since MAT is only accessing the women file at this stage, it will not detect any individuals below the age of 15. For example, if you specify an age bracket of 13-24, MAT will only consider a 15-24 age bracket.
Therefore, it is advisable NOT to set a placeholder for 15 since there are no data points below this age. NOT
If not considered, this will give a false impression that the indicator is capturing age brackets beyond the available data.
Select Source: Women

Variable: Age of woman

Click/switch on User Defined Value in order to set a placeholder:

Select Operator: Select less than or equal to

Select Default Value: 24 (15-24)

Set a placeholder: Since this is the first placeholder choose {1}

Click
Next, following the definition:
Any woman aged 15-24 gave birth before the age of 18
Similarly to the placeholder for the upper age bound, you can add a placeholder for age at first birth
Click on the green plus (+) sign to the right of the indicator to add an 'and' option

Select Source: Women

Select Variable: Age at first birth

Select Operator: Select less than since the definition states before the age of 18

Click/switch on User Defined Value in order set a placeholder:

Select Default Value: 18

Set a placeholder: Since this is the second placeholder select {2}

Click
Click the General Info tab

Scroll down to the Indicator Display, make sure to include the placeholders you set within the definition so you may later change the cut-off points in the MPI Central tab:

Click
As shown below, setting a placeholder will allow the user to choose the cut-off in the MPI Central tab

Educational Attainment Indicator (ALL - Placeholder/AND operator)
Click On
Name your indicator in the Title box and write the definition for your indicator in the Definition box. After naming and defining general information on the indicator, find the Rules tab to continue:

The definition states that the HH is deprived if ALL members of the HH aged 19+ have not completed secondary education.
As such, select the All option to match the definition:

Next, you want to input the Age variable from the definition:
HH is deprived if ALL household members aged 19+ have not completed secondary education.
Click on
Select the source: Individual

Select the variable: Age

Click/switch on User Defined Value in order to set a placeholder:

Select the operator: Greater than or equal to (+19)

Select value: 19

Set Placeholder: Since this is the first placeholder you have set, please select {1}

Click
The definition states that the HH is deprived if all household members aged 19+ have not completed secondary education . To implement this definition using the Indicator Builder's logic, we will deconstruct it as such: a variable for age (19+) AND a variable for years of schooling completed.
As such, you will want to add the AND operator.
Click on the green plus (+) sign to the right of the indicator to add an 'and' option

Now you will want to add the Years of schooling completed variable to account for secondary education (<12 years for secondary education)
Select Source: Individual

Select Variable: Years of Schooling, completed

Click/switch on User Defined Value in order to place a placeholder

Select Operator: Select less than (<12 years for secondary education)

Select Default Value: 12 (12 years for secondary education)

Set a placeholder: Since this is the second placeholder you have set, select {2}

Click
Find and click the General info tab

Scroll down to the Indicator Display and make sure to include the placeholders you set within the definition in order for it to later be changeable in the MPI Central tab.

Click
Placeholders allow the user to change the cut-off point of an Indicator in the MPI Central tab.

Cloning and Editing Pre-Set Indicators
In some cases, you may need to provide different proxies to the measure by slightly changing or adding to the definition. If you do not want to start from scratch, you may opt to use the cloning feature.
Improved Drinking Water Indicator (OR operator / Placeholder)
Consider the Access to Water indicator definition:
"HH is deprived if the household does not have any of the following sources: piped water into a dwelling, piped water into a yard or it uses bottled water."
In this example, the user may only select categorical variables from the drop down list, and the variables chosen will specify when a HH is considered deprived. However, here the user defined option is not applicable
Although this definition involves categorical variables only, in such examples, you may decide whether to give the user the option to choose a value. In order to do so, you must clone the indicator and consider adding another definition.
Consider an alternative definition where the round trip duration is part of the rule:
"HH is deprived if the drinking water source is not improved OR if the roundtrip to get drinking water is at least 15 minutes"
By adding the duration of the round trip to the definition, you have the option to allow the user to set a placeholder for the duration of the roundtrip through the user defined option.
The logic in this example is that the categorical variables are still the determinants of whether the household is considered deprived or not, however here we are adding another condition saying that the HH is also deprived "if the roundtrip to get drinking water is at least 15 minutes".
To begin please find the Improved Drinking Water indicator from the list of pre-set indicators:

Find and click the clone button to the right handside of the indicator:

A pop-up screen will ask you the following. Click yes!

Scroll down the list of indicators until you find the Cloned Improved Drinking Water indicator:

Find and click the edit button on the right side of the cloned indicator:

The following page will show the pre-set cloned indicator name and definition:


Since you are adding a new rule to the definition, rewrite your title and definition as follows:
Rename the title to: Access to Water (round trip)

Rewrite the definition to: "HH is deprived if drinking water source is not improved or if the roundtrip to get drinking water is at least 15 minutes"

Find and click the Rules tab

The rules tab will show the following:

The WS1 variable is applicable to the old definition, however you must incorporate the new definition. Let us look at the definition again:
"HH is deprived if the drinking water source is not improved OR if the roundtrip to get drinking water is at least 15 minutes."
As such, we need to add the "time to get water" variable, using the OR operator.
Click on to produce the OR
operator
Select the source: HH

Select the variable: From the drop-down list, select WS4: Time (in minutes) of roundtrip to get drinking water:

Select the operator: greater than or equal to

Select the value: 15 since the definition is at least 15 min

Click/Switch on User Defined Value since you will set a placeholder for the time in minutes:
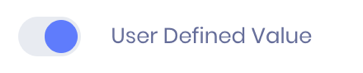
Select placeholder: Since it is your first placeholder for this definition, select {1}

Click
Find and click the General Info tab

Scroll down to the Indicator Display and make sure to include the placeholder you set within the definition.

Click
Placeholders allow the user to change the cut-off point of an Indicator in the MPI Central tab.

Glossary
Please refer to the glossary for reference to cross-cutting options


Data upload
1. Understanding the data structure
Creating a template file on Stata enables the Multidimensional Poverty Index Assist Tool (MAT) to understand the survey structure being uploaded by the user for a given country in a given year. The MAT can host various types of micro-data, such as household living conditions surveys, demographic health surveys, income expenditure surveys and labour force surveys.
Why we need to create a template file

A. Modules
The MAT can recognize five data files that will be connected to each other once signalled correctly by the data owner. The most general structure is as follows:
- Household file: mainly used to build assets and services indicators (mandatory)
- Individual Records file: mainly used to build education indicators (mandatory)
- Women file: mainly used to build women’s health and pregnancy indicators (optional)
- Birth History file: mainly used to build child mortality indicators (optional)
- Children file: mainly used to build child nutrition indicators, vaccination status, etc. (optional)
The MAT needs two mandatory data files to work properly - the household file and the individual file - while the other three files are optional.
To understand the five types of files, we make the following distinctions:
- The first file has information at the household (HH) level, i.e. one line per HH. Only one entry line per household can be added. This type of file will be referred to as the HH file in this manual.
- The second file has information at the individual level (HL). Several individuals may belong to the same household. This file allows multiple entries per HH, but only one entry per individual. This type of file will be referred to as the HL file in this manual.
- These two files are linked through the key variables explained below. These two files are mandatory for computation purposes.
- The Women file allows multiple lines per HH but one line per woman. The individual identification (ID) and HH ID in this file are linked to both the Household file and the Individual Records file, according to the key variables. This type of file will be referred to as the WM file in this manual.
- The Birth History file allows multiple entries per woman. These records may or may not be part of the Individual Records file (eg. a deceased child can be listed in this file, but not in the Individual Records file). In this case, the link between the files is done through the HH ID and the woman’s individual ID as key variables. This type of file will be referred to as the BH file in this manual.
- The Children file allows multiple entries per HH, listing all children living in that household. The children identification variable should match their individual ID in the Individual Records file. This type of file will be referred to as the CH file in this manual.
B. Unique identifiers and decomposition variables
All files are linked through a unique identifier, specific to each observation. The unique identifier can be collected in one or more variables (explained further in Step 4). These unique identifiers are also called key variables. Overall, the MAT needs a set of three key variables to operate correctly: the Cluster ID Number, the HH ID Number, and the Individual ID Number.
Note: If the Cluster ID is not available, the variable should be created prior to uploading the data and should be assigned the value of “1” across all entries. In other words, the entire dataset will be considered to belong to one big cluster.
The MAT needs additional variables that must be clearly signalled in the data structure to be able to generate automatic results and reports, such as decompositions by region, gender, etc. These variables should have standard names and will be discussed in Step 4. These variables are also called static variables.
C. Codebooks and data upload
For the MAT to recognize the labels of your variables, the data codebook has to be uploaded. This can be done easily and will be discussed in Step 8. This also allows the MAT to display clear quantitative data in terms of clean codebooks and clean categories within the variables.
To upload data to the MAT, the files type should be in Excel (.xlsx). Each data file should be uploaded in two steps:
- The data file (.xlsx).
- The codebook file (.xlsx).
Note: Kindly keep all labels within the template in the same language for optimal user experience.
2. Creating a template in Stata
To begin organizing your data according to the correct template, you need to create a new folder on your desktop and name it according to the country you are working on. This folder should contain all your data files. It will host the newly generated templates and codebooks that you will upload to the MAT.
Right click on the folder and select ‘Properties’. This will allow you to obtain its location. Right click to copy the file location.
Open a new Stata ‘.do’ file and begin by typing in the following, which includes the location of the country file you created:
clear
cd "COPY PASTE LOCATION HERE \ NAME OF DATASET/COUNTRY YOU ARE WORKING ON"
As a first example, let us consider the mandatory Household file (hh.dta).
Step 1. Remove unidentifiable characters
If the text of the survey is not in plain English, some encoding must be done to ensure all characters are considered.
Type or copy paste this into your ‘.do’ file:
Make sure unidentifiable characters are correctly encoded, for example French or Arabic letters.
unicode analyze hh.dta
unicode encoding set Latin1
unicode translate hh.dta
use hh.dta, replace
Once finished with this step, select everything you have written so far and run the '.do' file on Stata to ensure there are no errors.
Note: The same steps should be repeated for all other files. Programmers need to change the file name only.
Note: The two most important files are the HH and HL files, because these files contain the main key variables (Cluster ID, Household ID and Individual ID) in addition to static variables that need to be renamed in order to be recognized by the MAT.
Step 2. Export codebook before addressing missingness
To extract the codebook paste the codebookout command below into your template '.do' file:
Ensure to replace the texts in red with the country name, source file and year that you are working on in the code below.
codebookout "C:\Desktop\Mauritania\raw_codebookhh2011.xlsx", replace
Run the code in Stata to ensure there are no errors.
Note: The same steps should be repeated for all other files. Please ensure that when changing source files at a later stage, you change the name of the codebook in Excel to avoid overwriting it. Example: codebookout "C:\Desktop\Mauritania\raw_codebookhl2011.xlsx", replace.
Step 3. Drop string variables and integers
Type or copy-paste the following into your template '.do' file:
ds, has (type string)
drop `r(varlist)'
Run the code Stata to ensure there are no errors.
Note: The same step should be repeated for all other files.
Step 4. Key variables and static variables
The key variables are:
- IDCL for the cluster identifier variable.
- IDHH for the HH identifier variable.
- IDLN for the individual line number or individual identifier variable.
- BHLN for the child line number in the Birth file, the Multiple Indicator Cluster Surveys (MICS), or birth column number in the Birth Record file in the Demographic and Health Surveys (DHS).
The static variables are:
- RU is for the rural-urban variable.
- Governorate, when available, is the area to be represented on the country’s map.
- hhsex is for the gender of the HH head.
- hhweight is the sample weight variable.
- Gender is the gender of the individual.
- Relation is the relation to the head of HH.
- Age is the age of the respondent.
- hhs is the number of household members, also known as the household size.
If the following variables are available in the dataset, they should be specified as follow:
For now, you will need to:
- Make sure the names are the same. The order does not matter.
- When variables are uploaded to the MAT, the system will drop any underscore (_) and will convert all capital letters to lower-case letters.
- Pay attention to the variables names that could be uploaded with the same name (example: age1, Age1, AGE1, Age_1). MAT will prompt a “duplicate error” message.
- Missing values: IDHH and IDLN should never have missing values. The MAT will prompt an error message.
- The sample weight variable “hhweight” should not have missing values or 0 values.
- If the variable is not available.
(a) For Cluster ID: generate a variable with value 1.
(b) For RU or governorate: generate a variable with value 0.
Again, if the Cluster ID (IDCL) static variable is not available in your dataset, generate the variable with a constant value across all observations (either 0 or 1). The MAT will understand that there is no variability in that field and will not produce decompositions by this variable. All households have to have a distinct ID, and all individuals within an HH should have a distinct line number.
Example:
Let's take an example from an MICS survey:
- In the HL file, we may find that IDLN is the line number.
- In the BH file, we may find that BHLN is the birth column number and the IDLN in the BH file is the respondent’s line number.
In this case, we display two households from cluster 10. The first one has two individual while the second has four.
Let's take another example from the DHS survey:
In this case, we display two households. The first one has an individual (with line number IDLN=1) reporting one birth while the second has an individual (with line number IDLN=2) reporting two births.
The BH file is linked to the WM file and the HL file via IDLN. Births are not linked to other files except through the eligible woman responding.
To better understand the structure, some children from recorded births are alive and are thus recorded in the HL file and the BH file; others are deceased and are thus recorded in the BH file only and not in the HL file.
1. Rename static variables
Assume that in your data file you have the following variables:
- HH1 is the cluster number.
- HH2 is the household number.
- HH6 is the rural-urban variable.
- HH7 is the governorate variable.
- Sweight is the sample weight.
*If the cluster number is missing: generate a dummy variable taking the value of 1*
To rename them, copy-paste the following:
** rename static variables **
rename HH1 IDCL // cluster number
rename HH2 IDHH // household number
rename HH6 RU // rural / urban
rename HH7 governorate // governorate
rename sweight hhweight // household sample weight
order IDCL IDHH RU governorate
Main static variables should not have missing values, if there are missing values, the Excel data file will not upload to the MAT.
Household sample weights should not have a value of 0.
2. Rename labels for governorate names according to the MAPS Excel sheet
For some countries the maps cannot be displayed. Instead, results will be presented in a table based on the governorate names in the survey. The names of the country and governorates on the template must be identical to the names found in the Excel sheet for them to show correctly in the results maps on the MAT.
Link for the MAPS Excel sheet:
https://unitednations.sharepoint.com/:x:/r/sites/ESCWA-WG-MAT/Shared%20Documents/Country%20Cases/MAPS%20-%20Arab%20Countries%20Geo_RA-SS-v2.xlsx?d=wfb433425156d4c3d92ce98eafc8c853f&csf=1&web=1&e=NoCckA
Run the code in Stata to ensure there are no errors.
3. Drop incomplete interview
It is advisable to drop all incomplete interviews from the dataset so as to better reflect the missingness analysis in the MAT.
If the “Results of Interview” variable is result, and the complete interviews are coded with result=1, you can type the following in Stata:
** drop incomplete results **
drop if result != 1
Step 5. Clean labels for no response
This is a crucial step for the correct identification of deprivation and non-deprivation.
Labelled variables have a different set of categories. Some labels will indicate deprivation, while others will indicate non-deprivation. A third category will indicate not belonging to either identification.
In this step, you are looking for answers in the codebook such as “I don’t know”, “DK”, “not sure”, etc.
It is important to signal such categories to the MAT as a category that is missing valuable information, because as you have seen in the “Indicator Builder” manual, the MAT expects the user to select the labels that define deprivation, while other labels will be identified as non-deprived by default. So, if the “DK” category, for example, was not selected in the definition of deprivation when building the indicator, the MAT will consider this category as non-deprived by default. Such categories do not offer valuable information for identification and could be considered missing.
In summary, the objective of this step is to replace these variables with missing “.” to make sure the category is not confused with a non-deprived status.
To do so, start by inspecting the codebook that was created in your country file and check the different versions of labels that offer no valuable responses to the deprivation status.
Let us assume that in your codebook you have only two missing labels: “I don’t know” and “DK”.
In the template ‘.do’ file you may name this section:
**clean non-response**
Option 1: Use this option to clean your labels if all variables have the same code for the modality of the label.
For each of your missing labels you must copy-paste the code below and replace the text in red with your missing label, as copied from the codebook. Let us assume your missing label is “I don’t know”.
For each var of varlist _all {
replace `var' = . if `var' == "I don’t know":`var'
capture labvalch3 `var' ,subst("I don’t know" "")
}
Repeat this for every missing label. In this second example let us assume your missing label is “DK”.
For each var of varlist _all {
replace `var' = . if `var' == "DK":`var'
capture labvalch3 `var' ,subst("DK" "")
}
Option 2: Use this option if each variable has a different code for the modality of ‘don’t know’:
*Replace the red text with your relevant information.
Replace variable = . if variable == code of the ‘dont know’
Now you should define the new label for the code of missing with blank.
Label define LABEL old_code_of_missing “”, modify
Example: Using variable hv803 //‘DK’ // code for DK is 8. We want to replace the ‘8’ with a dot which will be read as blank. It will show as follows:
Replace hv803 = . if hv803 == 8
Label define HV803 8 “”, modify
Run the code in Stata to ensure there are no errors.
Note: The same steps should be repeated for all other files.
Step 6. Generate adjusted variables to account for survey design missingness
In this step, you will need to adjust variables that are not requested of all the individuals, based on their previous answers.
Why do we need to adjust for this type of missing values?
These values are known but not recorded to avoid redundancy. The three scenarios below illustrate such redundancies.
Scenario 1: When measuring the number of births/deliveries, some surveys will not ask women who were never married about the number of deliveries they have had. Instead, they will be reported to have missing values in the variable of interest. However, by design, the number of deliveries for non-eligible women is assumed to be zero. In this case we create an adjusted variable that would replace the missing values of non-eligible women with zero.
Scenario 2: For education, if a person did not go to school, they are not asked the question of highest educational attainment (level/grade). By survey design, it is assumed that a person who never attended school has zero years of education. As such, the years of education variable should be adjusted by re-coding it to zero for this category of respondents.
Scenario 3: At the household level, some surveys ask about the source of electricity through a sequence of variables. For example, it may start by asking if the household has “electricity” (yes/no). If yes, the household is posed a series of other questions about the source, “public network” (yes/no), “private generator” (yes/no), etc. These prompted questions are not asked of households that do not have electricity. They are thus reported missing, while they can be recorded as “NO” by default.
Missing values would result in excluding households from the analysis, and we would like to maximize the use of the data from a representative survey. The objective is to reduce as many exclusions as possible. We can illustrate the effect of a missing observation on the deprivation status of an individual or a household in a given indicator.
If you refer to the “Indicator Builder” user manual, at the Composite Indicators Section, you can see the table below illustrating the effect of missing values of the variables on the deprivation status in the indicator. An indicator usually defines deprivation at the household level, based on the deprivations of its members. It can be built using one or more variables. A missing value in one of the variables often results in a missing deprivation status.
The following table, extracted from the Expansion Section of the same manual, illustrates the effect of missing indicators for one household member on the deprivation status of the entire household, where one missing value could exclude the entire household from the analysis.
To reduce missing values and exclusions, some variables can be simply adjusted, based on a priori knowledge of the survey design.
To generate adjusted variables, please consider the following examples from the Iraq 2018 MICS dataset.
Example 1 **adjusted attendance**
In this example, we need to adjust the data for missing values that are due to survey design. To do so, we start with exploring the definition of the indicator of interest, which is “School Attendance” in this case, and determine the variables needed to define deprivations.
Definition: HH is deprived if any child in the household aged 6-18 is not attending school and has not completed secondary education.
To define the deprivation, three variables need to be considered: The first variable to be considered is age (6≤age≤18). The second variable is school attendance (ED9). The third variable is years of schooling (<12years).
Once the variables are defined, we explore the frequency of their missing values. As illustrated in the Stata output below, ED9 has 77,000 missing values. It is suspected that such high frequency of missing values is due to survey design. The questionnaire should explain the logic of the survey design. For example, like Scenario 2 above, persons who never attended school will not be asked about their current attendance.
In the Iraq 2018 MICS questionnaire (see extract below), variable ED4 “Ever attended school or any early childhood education programme?” is a question that terminates the interview on the Education Module if the answer is “NO”, and the interviewer will move to the next line. This means that all individuals that answered “NO” on ED4 will have missing values for the rest of the questions in this module. Similarly, answering “NO” on ED8 also terminates the interview.
Alternatively, some variables may request interviewers to skip some questions without terminating the interview. For example, all individuals answering “NO” on ED12 about “school tuition support” will skip question ED13 on “the provider of the tuition”, thus their answer on ED13 will be reported as missing.
Consequently, the 77,000 missing values in ED9 could be due to the ED3, ED4, ED7 or ED8.
To understand the nature of the missing values, one may tabulate ED9 with the preceding variables identified from the questionnaire.
As shown in the Stata output below, ED8 is a verification question and seems to have the same number of missing values as ED9. We continue with ED7, the age-eligibility question, where 41,708 were either less than 4 years old, or above 24 years old, thus not asked about their current enrollment. This leaves us with 35,392 missing values. So, we continue the investigation.


Another tabulation between ED9 and ED4 shows that only 9,645 observations were not recorded as they are below the age of 4 years (as shown in the tabulation with ED3). The rest never attended school or an early childhood education programme (25,731 observations) or did not respond to ED4 (16 observations).


Now that the source of missingness is clear, one may adjust ED9 after understanding the codebook for this variable.
The codebook shows that “NO=2” for ED9.
clonevar ED9_adj=ED9
replace ED9_adj=2 if ED9==. & ED4==2 // to account for those that never went to school (25,731 real changes made)
replace ED9_adj=9 if ED4==9 // no response (16 real changes made)
replace ED9_adj=3 if ED3==2 // below the age of 4 years (9,645 real changes made)
replace ED9_adj=4 if ED7=2 // above the age of 24 years (41,708 real changes made)
label var ED9_adj "Attended school during the current year of the survey"
tabulate ED9_adj,m
Categories 3 and 4 can be labelled as “below 4 years old” and “above 24 years old”, respectively.
Example 2 **adjusted ever gave birth**
Another example is about missing values in the “giving birth” variable. In this example, only the direct code is given below. The questionnaire exploration and other tabulations will be left for the users.
clonevar CM1_adj=CM1 // delivered (1=yes, 2=no)
replace CM1_adj = 2 if MA5==3 // never married will not be asked cm1, so adjust missingness
replace CM1_adj = 1 if CM11>=1&CM11< // CM11= number of children ever born
label var CM1_adj "Ever given birth, adjusted for never married women"
Run the code in Stata to ensure that there are no errors.
Note: The same steps should be repeated for all other files and variables that may have a large number of missing values to account for survey design missingness.
Step 7. Generate variables (referring to the ‘.do’ file)
Here you will need to generate variables that are not found in the data file but that are required to build indicators for your selected MPI framework.
The list below entails the usual variables that need to be generated prior to uploading the data, as the MAT v2.2 cannot perform mathematical operations on variables such as multiplying, dividing, adding or subtracting variables.
*Crowding *Schooling years *Grade level *Education gap
For your template ‘.do’ file, follow some of the examples below from the Revised Arab MPI framework in order to generate the variables:
Example 1: **Crowding**
The crowding variable is the average number of persons per room in a household. The crowding variable is used to define deprivation in the overcrowding indicator where a household is considered deprived if it has more than “x” number of people per sleeping room. According to the Revised Arab MPI framework, the threshold is three persons or more. To define this deprivation, an interim “crowding” variable is needed. This variable can be computed by dividing the “Household size” variable over the “Number of rooms in the household” variable.
If the value of “crowding” is three or more, then the household is considered overcrowded, thus it is deprived.
Some variations may apply with the definition. For example, one may consider persons aged 5+ or 10+ in the numerator, or one may consider the “number of sleeping rooms” in the denominator. The numerator can be computed by taking the difference between the household size and the number of children below 5 years of age.
The code in Stata can be as follows:
gen age5p = HH11-HH14 // generate numerator “age5p”=”household size”-“number of children under 5”.
gen crowding = age5p/HC2 if HC2<. // number of household members 5+ divided by the number of rooms.
// The deprivations rule is as follow: a household is deprived if the average number of persons per room is three or more. To have an idea on the deprivation rule that you can build in the MAT, you can run the following code in Stata.
gen overcrowding=(crowding>=3) if crowding<. // number of persons 5+ should be>=3 per room.
Example 2: **Schooling years (grade completed)**
The schooling years indicator counts the number of completed years of formal schooling. It ranges from 0 to more than 16 years for postgraduate studies. It uses the following variables:
ED6: Highest grade completed at that level (1=yes, 2=no).
ED5B: Highest grade attended at that level.
ED4: Ever attended school or any early childhood education programme (1=yes, 2=no).
ED5A: Highest level of education attended.
This variable is usually used in the “education attainment” indicator, where a household is considered deprived if no adult has completed “x” years of schooling. In the Revised Arab MPI framework, this threshold is 12 years of schooling per adult.
The schooling years variable can be computed as follow:
gen schooling_years=ED5B if ED6==1 // if grade is completed
replace schooling_years=ED5B-1 if ED6==2 // if highest grade attended is not completed
replace schooling_years= 0 if ED4==2 // never went to school.
replace schooling_years=0 if ED5A==0 // kindergarten
replace schooling_years= schooling_years+6 if ED5A==2 // intermediate = ED5B+6yrs of primary
replace schooling_years=schooling_years+9 if ED5A==3 //diploma=ED5B+(6 primary+3 intermediate)
replace schooling_years=schooling_years+6 if ED5A==4 // secondary = ED5B+9
replace schooling_years=schooling_years+12 if ED5A==5|ED5A==6 // bachelor
replace schooling_years=schooling_years+16 if ED5A== 7 //postgraduate
replace schooling_years=. if ED5A==.&ED5B!= //grade known, but level unknown should be missing
replace schooling_years=ED5B-1 if ED6==. // grade known, completion unknown, downgrade 1 level
label var schooling_years "Years of Schooling, completed"
Example 3: **Grade level**
The Grade level indicates the currently attended year of schooling. This indicator is a combination of grades and levels. It uses the following indicators:
ED10B: Grade of education attended current school year. ED10A: Level of education attended current school year.
It is usually used to compute the education gap variable and then the age schooling gap indicator, where a household is deprived if any child is two or more years behind their appropriate grade, as per the Revised Arab MPI framework.
The grade level can be computed as follow:
gen grade_level=ED10B
replace grade_level=0 if ED10A==0 // maternity
replace grade_level=grade_l+6 if ED10A==2 // intermediate
replace grade_level=grade_l+9 if ED10A==3 // diploma, after intermediate
replace grade_level=grade_l+6 if ED10A==4 // secondary, add 6 yrs of primary
replace grade_level=grade_l+12 if ED10A==5|ED10A==6 //dimploma, bachelor
replace grade_level=grade_l+16 if ED10A==7 // post-graduate
replace grade_level=. if ED10A==.&ED10B!=. // grade known, but level unknown should be missing
Example 4: **Education gap**
The education gap variable shows the number of years a student is behind their appropriate grade. This variable is usually used to compute the age schooling gap indicator, as mentioned in Example 3 above.
To compute the education gap variable, take the difference between the actual age and the appropriate age.
education gap = actual age - appropriate age
To complete the computation, the “appropriate age for a grade” will be calculated by adding 5 years to the grade, because the starting age of school is 6 years. For example, for the first grade “grade_level=1”, the appropriate age for grade 1 is 6 years old. So “appr_age=grade_level+5”.
In Stata the variables can be coded as follow:
gen appr_age=grade_level+5 // appropriate age
gen educ_gap=HL6-appr_age // difference between actual and appropriate age
label var educ_gap "Difference between appropriate age for a grade and actual age of student"
Run the code in Stata to ensure there are no errors.
Step 8. Export data and codebook to Excel files to upload
Note 1: Before exporting your data, make sure all variables are labelled.
Note 2: In the codebook, check variables only have the following:
(a) Numeric (b) Double (c) Number NOT→Integer
On your template ‘.do’ file, you may name this step: *Export data and codebook to upload*.
Create a new folder within your old country folder and name it: files-to-upload.
Right click on the new folder, find properties and copy the location link.
To export the data into Excel, use the “export Excel” command. To export the codebook, use the “codebookout” command.
Copy- paste the following code into your template ‘.do’ file, ensuring to replace what is in red accordingly:
export excel using "your location link\files-to-upload\hh.xlsx", firstrow(var) nolabel replace codebookout "your location link \files-to-upload\codebookhh2011.xlsx", replace
Once step 8 has been completed, you may now move on to a new source file and repeat all eight steps within the same ‘.do’ file. Ensure that when changing source files at a later stage, you change the hh within the link, so your newly exported files do not over-write the old ones. For example, for the HL file you can use:
codebookout "C:\Users\10176021\Desktop\Mauritania\ files-to-upload\codebookhl2011.xlsx", replace.
Run the code in Stata to ensure there are no errors.
3. Upload data to the MAT surveys tab
In the MAT, find and click the surveys tab and select the country and year to which to you want to upload data. You may find the page below for Algeria 2012:
To upload a data file, click on the blue button. To upload a codebook, click on the yellow button. In both cases, a pop-up window will give you access to your saved files, which you can browse and then select the relevant folder to pick from.
Once you upload your surveys and codebooks, please ensure that the number of clusters, HHs, individuals, and the number of variables all match your original data set. An example is given in the screenshot below.
For any further assistance, questions or comments please contact ask.mat@un.org.